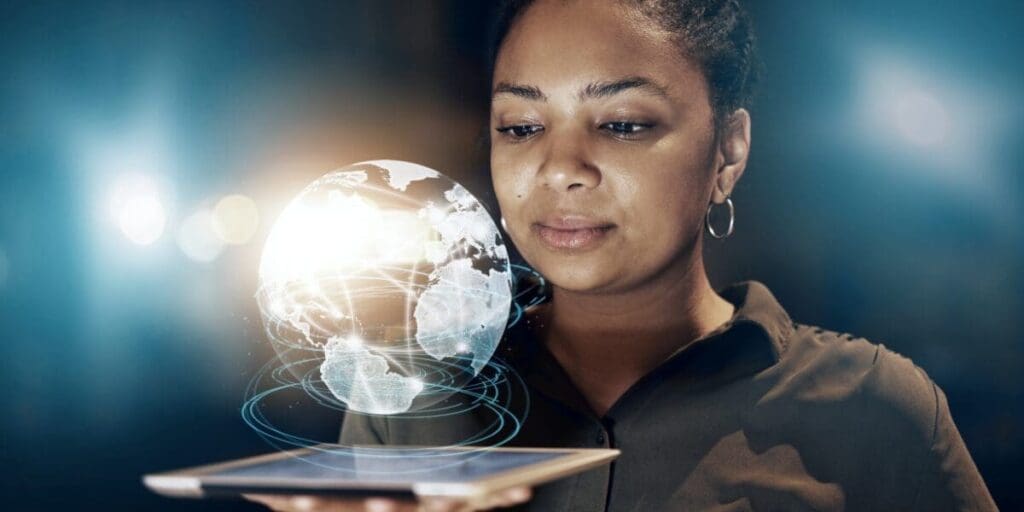
Understanding AI in Project Management
Artificial intelligence (AI) is all the rave. Our organization has been using AI for more than a year. What we can say is that it is a mixed bag. You will be severely disappointed if you think AI will be a panacea for individual thinking and creativity.
You may have seen Google’s Gemini AI in the news. The quality of AI depends on an algorithm and data sources, which are often unknown to those using the system. However, if we make AI systems unique for our company, pointing to data sources and using known algorithms, they will become more usable and effective.
AI operates through complex algorithms and data analysis to simulate human intelligence in machines. At its core, AI involves the creation of machine learning (ML) models, which are trained using vast amounts of data. These models learn to recognize patterns, make predictions, or perform tasks by analyzing the training data. The training involves feeding the model with input data, processing it through multiple layers of algorithms (often neural networks), and adjusting the model’s parameters based on its performance in making accurate predictions or decisions. Over time, and with sufficient data, AI systems improve their accuracy and efficiency in tasks such as speech recognition, image analysis, decision-making, and natural language understanding. AI’s adaptability allows it to be applied across various domains, from healthcare diagnostics to autonomous vehicle navigation, enhancing its utility and transformative potential in numerous fields. [Source]
Projects are executed in various domains, but the common thread is that they are not operations; therefore, there are plenty of unknowns. A large language model and machine learning can help us learn even before we start working – within parameters.
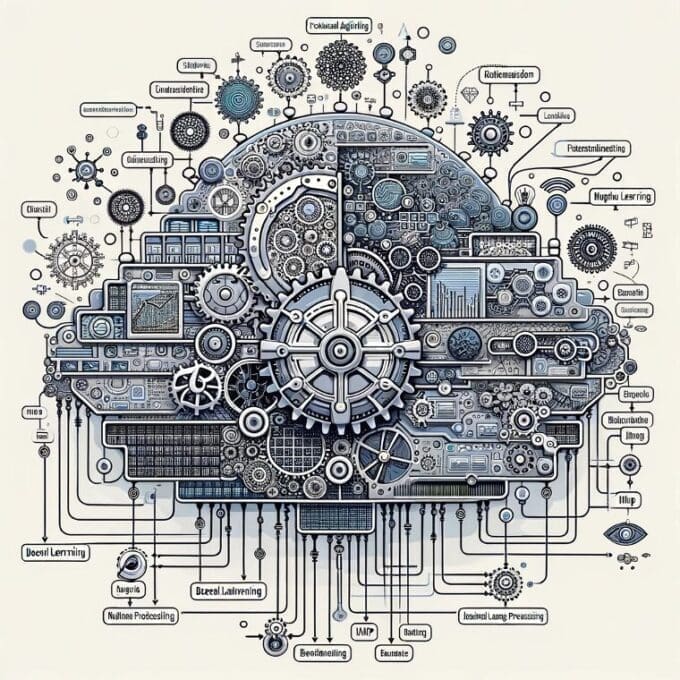
The Cornerstone of Effective Use of AI Tools
The cornerstone for the effective use of AI is data. One old saying in technology is “Garbage in, Garbage out.” To that end, the data must be high quality and appropriate to what one hopes to achieve. It must also be continually collected, updated, and secured. When Open AI launched ChatGTP2.5, it came with a warning that the data was only current to 2021. Another “gotcha” is that the responses can be far afield from the query. In earlier versions, we used it for book research and found the information from the prompt was way off base. If one did not know the topic, the response might appear valid, but from an expert perspective, it was far from it. In this way, the reviewer must be competent in the topic.
The foundation supporting this cornerstone is that an organization must be ready for AI. Somehow, all historical data from projects, documentation, metrics, risk analysis, organization goals, and monitoring, external data (e.g., global impacts) procedures for privacy and security, must be collected in a form that will be accessible to the AI. This data is a good starting point, and every single category may not be required.
Working with various AI chatbots that are currently available, another part of the foundation for effective use is what is termed “Prompt Engineering,” which means asking the right question the right way. Asking, “Please give me data on Project X,” may get you data, which probably won’t be what you hoped to get. Improving the prompt would look more like, “Please provide the risk register, risk analysis, mitigation strategy, and risk cost impacts from Project X. Please provide in text format and highlight all cost impacts.” Prompt Engineering training may be necessary. We are imperfect and have learned the hard way to be as specific as possible.
Uses for Predictive Analytics
Using AI to analyze an organization’s project management processes and historical project data (including project plans, records, risks, outcomes, budget, and schedule) can have significant predictive implications that enhance project success. One outcome of utilizing AI in project management is that it removes the biases that sometimes direct specific actions on a particular path.
Here are eight key areas where AI can make impactful predictions.
1. Project Success Prediction
AI can analyze historical data to predict future project success rates based on project type, team composition, budget allocations, and timelines. It identifies patterns and trends from past projects, providing insights into what made certain projects successful or unsuccessful.
2. Budget and Cost Overruns
By examining historical budgeting data and outcomes, AI models can predict potential budget or cost overruns for new projects. These predictions help organizations allocate resources more effectively and implement strategies to mitigate financial risks early in the project lifecycle.
3. Schedule Predictions
AI algorithms can forecast project timelines with greater accuracy by learning from past project schedules and their adherence or deviations. This capability enables better planning and time management, reducing the risk of schedule slippage.
4. Risk Identification and Mitigation
AI can analyze historical project records to identify risks that were not anticipated but significantly impacted project outcomes. By learning from these instances, AI can highlight potential risks early in new projects, allowing teams to develop mitigation strategies proactively.
5. Performance Optimization
AI can recommend process improvements and optimizations by analyzing past project performance. It can suggest adjustments in project management practices, resource allocation, and workflow optimization to enhance overall project efficiency.
6. Resource Allocation
Through analyzing past project data, AI can predict the optimal allocation of resources (human, financial, technical) for future projects, leading to improved utilization and productivity.
7. Quality Control
AI can help predict quality-related issues by analyzing defects or issues encountered in previous projects. This predictive capability allows for implementing quality control measures early in the project lifecycle to minimize the impact on the final product.
8. Implementation Considerations
While AI offers significant advantages, successful implementation requires quality data and thoughtful integration into existing project management processes. Organizations must ensure that historical data is accurate, comprehensive, and digitized in a format that AI algorithms can process. Additionally, there needs to be a cultural shift towards data-driven decision-making, supported by continuous training and development for project managers and teams to leverage AI effectively.
Conclusion
This article is going to be divided into two and perhaps three portions, so stay tuned for future publications. The main takeaway from part one is that, at this point, the potential for AI to revolutionize project management hinges on its seamless integration with existing systems and workflows. This integration enables project teams to leverage AI tools for predictive analytics, risk management, and resource optimization without disrupting their established processes. Achieving this harmonious integration, however, requires a strategic approach. There will be more on applications and some other topics in the next two articles.
Upcoming Live Event
On Wednesday, March 20, at 12:00pm EST, Erik van Hurck will guide you through the Copilot studio and will show you the chatbot’s capabilities in combination with AI. Supercharge your Project Management Office by giving it an extra pair of “digital eyes, ears and hands.”
Live Event: Copilot Studio and the PMO – Creating a Capable and Useful AI Assistant
Wednesday, March 20, 12:00pm – 1:00pm EST
Earn 1 PDU: 1 Ways of Working
Click to register now and join us live!
Elevate your project management skills and propel your career forward with an MPUG Membership. Gain access to 500+ hours of PMI-accredited training, live events, and a vibrant online community. Watch a free lesson and see how MPUG can teach you to Master Projects for Unlimited Growth. JOIN NOW
Michael Shost, PMI-PMP, PMI-ACP, PMI-RMP, CEH, SPOC, SA
Dear MPUG community,
The article highlights the cornerstone of effective AI use: high-quality, appropriate data that is continually collected, updated, and secured. Indeed, as I have seen in my work at the vanguard of AI in PMOs and project management, data readiness is crucial for organizations seeking to harness the power of AI.
Moreover, the author’s emphasis on “Prompt Engineering” resonates deeply with my experience. Crafting the right queries is an art that requires both domain expertise and an understanding of AI’s capabilities and limitations.
The eight key areas outlined for AI’s predictive impact are spot-on. I would further underscore AI’s transformative potential in risk management, where machine learning algorithms can uncover hidden patterns and dependencies, enabling proactive mitigation strategies that dramatically improve project outcomes.
As we continue to push the boundaries of AI in project management, it is crucial to approach integration strategically, as the author rightly notes. Beyond technical considerations, this also means fostering a culture of data-driven decision-making and continuous upskilling.
I eagerly await the subsequent parts of this series, which promise to delve deeper into applications and other critical topics. This article serves as an excellent foundation for the exciting discussions to come.